It’s easy to see why AI seems to be taking over the headlines. Even casual experimenting with the latest AI models such as Claude 3, GPT 3, or 4 shows they are very good at conveying knowledge, a skill that will surely give them a place in today’s world.
However, at the same time, there are clear limits to these models. These limitations are that any model can only work within a well-defined and limited knowledge base. Claude 3, for example, can comment on topics ranging from philosophy to chess. But playing chess or a basic understanding of the game is beyond its skill set. Similarly, questions about how philosophical concepts can be applied are beyond its scope.
“An amusing interchange came when I asked the chat box whether it thought it was conscious. The answer was no because computer programs do not have inner states of consciousness.”
Panpsychism and AI
Well-known philosophers such as Thomas Nagel favor a theory known as panpsychism, which argues that consciousness is a foundational property of the universe represented to various degrees in all material objects. Claude 3 was familiar with Nagel, meaning every printed word was in Claude’s database. Yet, Claude could not connect concepts within the database beyond relating a question to the literal expression of an aspect of the database.
Now, I can hear you saying that these models are only the beginning of our AI adventure, and it’s only a matter of time before these limitations fade into the past. Unfortunately, it is not that simple. Limits on essential resources define the fundamental limitations. We need vast resources, including energy, minerals, and metals, to scale these models to larger knowledge bases and more complex tasks. By and large, we conflate the potential of AI with its ability to solve the critical resource shortages that the world faces, which are perhaps the most imminent existential issues humanity faces. Now, you can rightfully reply that we have heard these same arguments regarding the proliferation of tech. There were arguments, for example, that the cloud would overwhelm our ability to produce energy. Those arguments – at least so far – have not come to pass.
Artificial “General” Intelligence
Albeit the essence of AI and its proponent’s belief in its transition to artificial general intelligence (AGI), something very similar to human intelligence, adding new information to a database will result in exponential growth.
The size of the cloud, IoT, and even transistors evidences linear or, more generally, polynomial growth. This means that more enormous clouds, for example, increase resource needs proportional to their additional size. Expanding the cloud size tenfold would increase resource needs equally by tenfold.
One exception might be manufacturing transistors, in which smaller chips lead to resource needs increasing exponentially faster than the size reduction; halving the size leads to a 32-fold increase in resources while reducing the size by a quarter would lead to more than a thousand-fold resource gain. However, these exceptions have been manageable thus far, as smaller chips lead to more significant energy savings than larger chips.
AI is a different animal altogether. Almost all attempts to improve AI will lead to exponential gains in resources. These gains may be offset by developing better techniques for training and using AI. Eventually, however, the implied resource increases will be so significant that techniques cannot compensate.
Consider chess, for example. On any one move, a player has 25 choices. This means that a chess player analyzing the game one move forward involves assessing 625 positions. Regarding two moves, about 390,000 possible positions must be evaluated. Looking ahead, four moves and the number jumps to 1.5 billion. Looking ahead, 30 or 40 moves would involve analyzing a number greater than all atoms in the universe. Of course, some techniques can reduce these extraordinary numbers. However, even the most robust AI chess computer will need help going farther ahead than ten moves. Although ten moves are enough to assure that computers can beat humans, there are still many positions where humans find better moves than the best AI analysis.
Additionally, chess puzzles are beyond any computer’s ability to solve, whereby humans can find solutions. A well-known example involves Mikhail Tal, whose pensive ten-minute walk resulted in a solution that no computer can solve from scratch. Then, there is the board game Go, which many consider more challenging than chess. In 2016, AI, bearing the sobriquet Alpha Go, made headlines by beating the world champion. By contrast, there were no headlines when a human player – well below the highest rated, defeated the Alpha Go in 14 out of 15 games.
The central point is that using today’s computers, it’s unlikely that either chess or Go will ever be solved, which means determining whether perfect play results in a win or draw. It is a computational question, but the number of computations that would be necessary is far beyond today’s largest computers.
Now consider that chess and Go are just two out of thousands, maybe millions, of domains to which AI can be applied, defined in terms of exponential growth. The critical point is that finite resources limit AI in ways that have not been applied to other technologies.
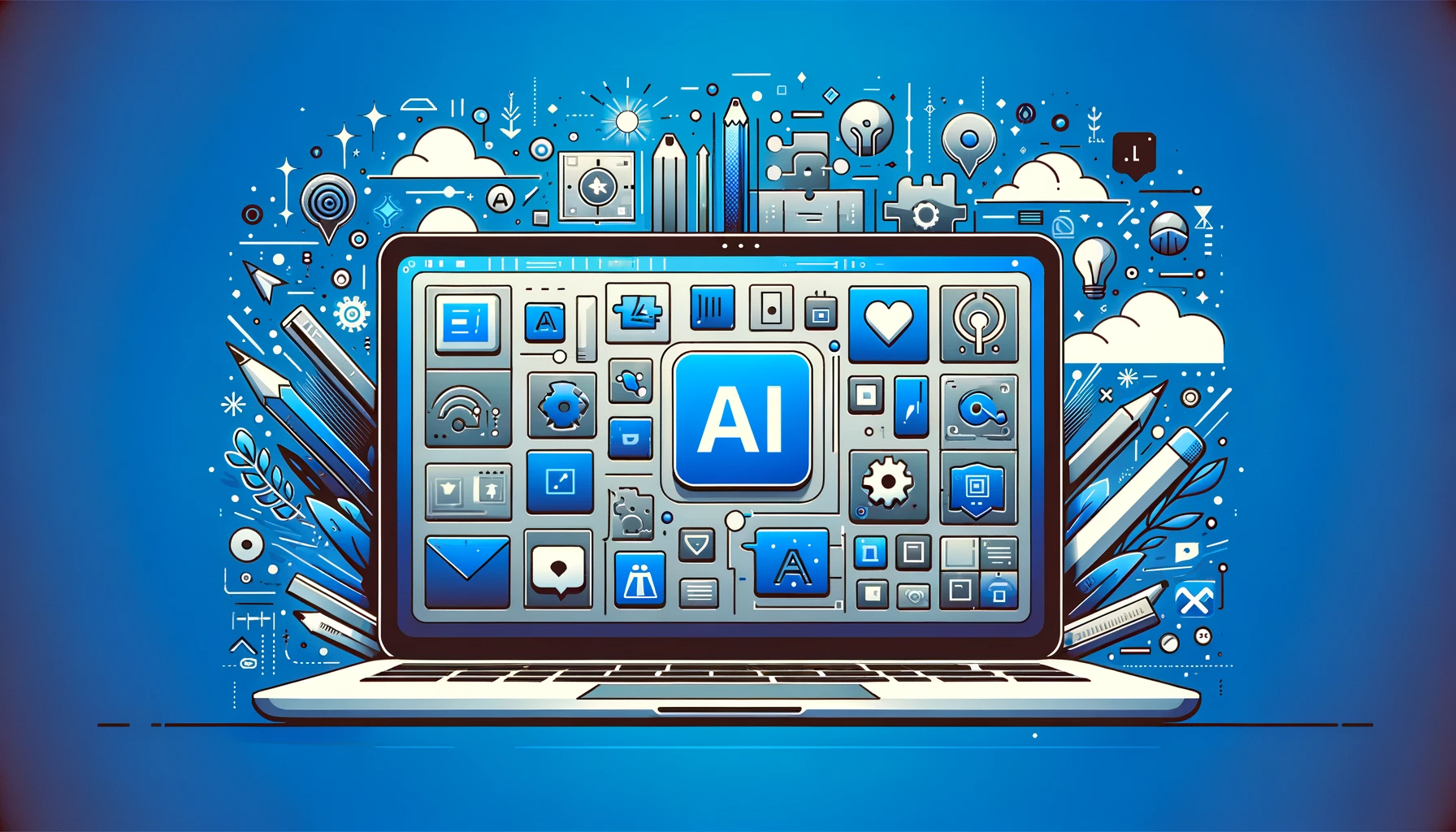
Critical Resources For AI
Our brief analysis yields two major interrelated conclusions. The first and broader reason is that for AI to play a more significant role in today’s world, the distribution of resources must become a primary concern. This necessitates cooperation with the Global South/East. Why?
In addition to their massive energy reserves, which include not only fossil fuels but minerals and technologies necessary to produce sustainable energies, the Global South/East has a preponderance of non-fuel minerals critical for our electronic and green technologies, which, according to the USGS, the U.S. is more than 50% net import reliant.
Folks, that’s not good news for the United States!
Canada, China, and Russia are the three most important countries that possess vital minerals for which AI and other technologies will create explosive demand in the future.
AI, specifically, as mentioned, though better techniques and algorithms will not solve the resource problem, they can facilitate it. In this case, cooperation between China, the U.S., and even Russia could produce the kind of technological improvement that would stretch the boundaries of what we can do with AI. We do not doubt that if we can stretch AI to the limits of what can be done with mutually developed techniques and a near-optimal use of resources, it could be enormously helpful in further stretching its capabilities – which, in addition to better techniques, could also include means of securing vast quantities of additional natural resources from both ocean and space exploration.
“There is a powerful argument for cooperation among mathematicians and computers of all nations to use AI as a powerful tool to keep the human race from veering on a path of inextricable extinction.”
Investing In AI
In the meantime, AI plays confined to relatively small but critical domains are the best bets. In large domains like language, the number of parameters or probabilities of predicting that define the state of the training can easily reach multi-trillions. The estimated costs of training a model for a large domain can run into hundreds of billions of dollars, and hideous amounts of energy are consumed.
By restricting the size of the small domain, you not only deal with lower costs but also have room for stretching outgrowth to many years of relatively steady growth rather than a few of explosive growth that sharply tapers off. Many companies fit the bill for using AI in relatively limited domains.
An example of a company that follows this path is the giant Japanese company, Hitachi. And there are many more. Another company that has recently gathered all the headlines and purportedly the most valuable stock on the NYSE is Nvidia, producing giant chips, meaning perhaps hundreds of millions of transistors used to train AI. Nvidia jumped into the competition because of its expertise in the gaming industry, where speed is required. It is a one-trick pony in the chip universe in which other chips have to work alongside GPUs to manage the flow of information.
This “competitive” edge is highly dependent on the company’s ability to innovate well beyond the simple speed of GPU, whose potential, thanks to exponential growth, will be most spent within the next two years. This exhaustion will then favor companies whose chips can deal with the fantastic amounts of energy required by massive chips. Currently, Advanced Micro Devices, with its chairman, broke new ground in energy efficiency with her Ph.D. in energy efficiency and semiconductors.
The need for cooperation is best illustrated by the Chinese company Alibaba. It is likely that BABA cannot use the sheer power of Nvidia with its chips and certainly had nothing similar to what Nvidia has today when it introduced AI training programs with 10 trillion parameters. (Note each parameter measures the probabilities of where electrons are very narrow time limits). Today, for Nvidia, 10 trillion is still an aspiration, which shows how vital cooperation can be in developing the techniques that can keep the AI bandwagon from petering out and, with it, a hope for human staying power.
Final Thoughts…
The message, as we have continued to stress in these blogs, is that cooperation is a means to helping the world survive. Yes, our power makers may – think America is exceptional – whether it is or not is a topic for a forthcoming blog. Still, at this point, our insistence on domination will very likely mean that even in a best-case scenario, we will dominate nothing. We don’t have to love those with whom we cooperate, but we must accept that working together not only benefits both of us but also all of mankind.